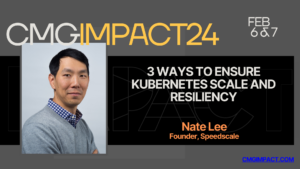
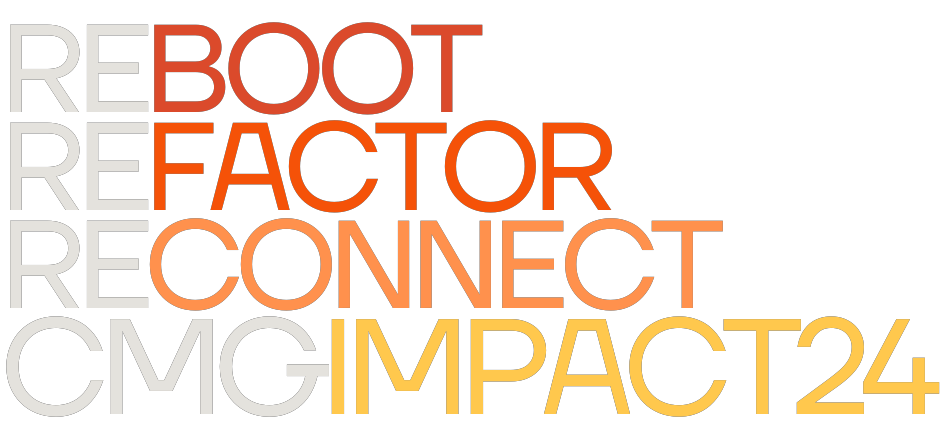
FEB 6 & 7
ATLANTA, GA USA
CMG IMPACT is your source for education and peer-to-peer exchange of all things digital transformation, enterprise IT, and infrastructure.
- 00Days
- 00Hours
- 00Minutes
- 00Seconds
2024 Partners
MULLEN FOUNDATION
What to Expect at IMPACT24
High Value Learning
Keynote and breakout sessions explore major trends in enterprise IT and digital transformation
Tech Demonstrations
Key opportunities to learn about real solutions and product offerings.
Networking & Exhibitor Sessions
Meet our speakers, sponsors, and other attendees through networking opportunities
Great Speakers
With an emphasis on peer-to-peer learning, attendees will learn actionable solutions from other industry experts
Exciting Giveaways
Participate in games, interactive sessions, and contests to win exciting giveaways
Live Learning
Engage in sessions at our in-person event in Atlanta
Ready?
Atlanta Sessions
We're now working with partners on bespoke sponsor participation packages.
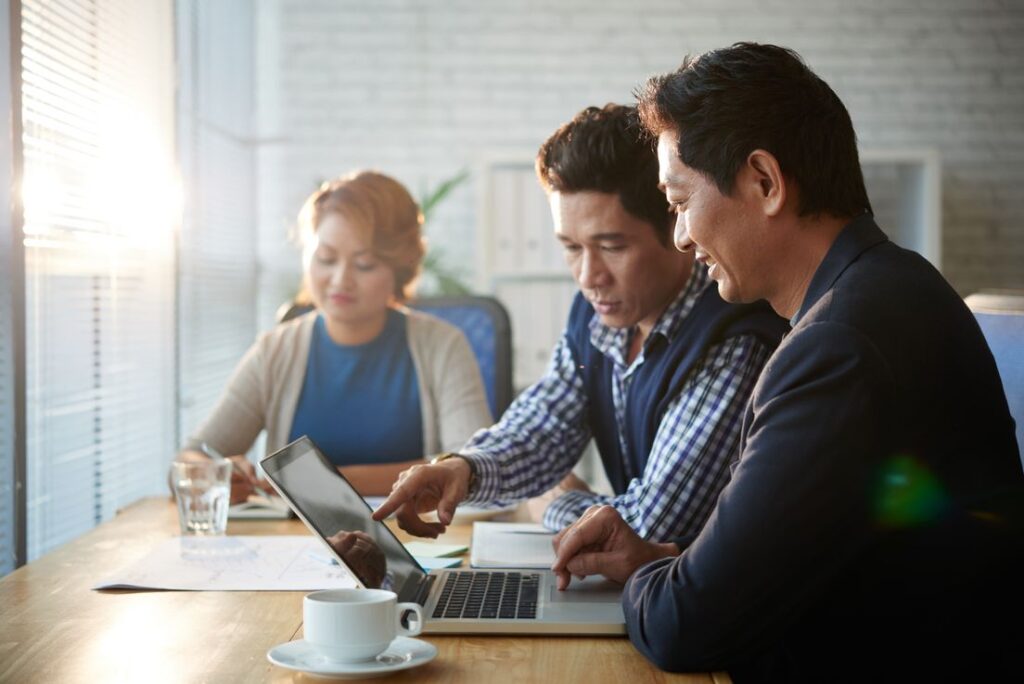
Let's Reconnect in Atlanta
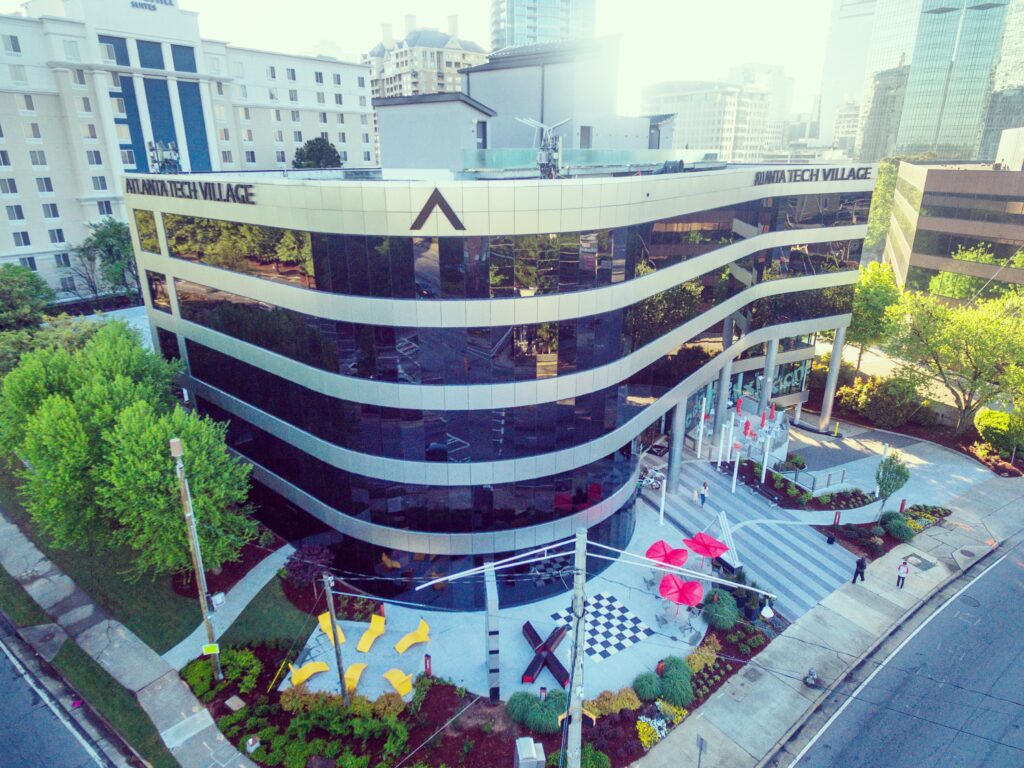
IMPACT24 will be held in Atlanta’s premier business district, Buckhead, home to a growing number of innovative technology companies, and a hub for commercial real estate and finance. Located in the geographic heart of metro Atlanta, Buckhead is an address of both distinction and convenience.
Atlanta Tech Village is a technology incubator complex founded by David Cummings in 2012 as a small business incubator. The Village is now the fourth largest tech hub in the US, housing more than 300 companies, and partners with larger corporations like Turner, Google, Amazon, Microsoft and Coca-Cola to form relationships between startups and larger establishments. Past companies have included Yik Yak, BitPay, and IO Education.
The village has about 1000 entrepreneurs working in the complex, which are connected to angel investors and funding sources, in addition to business mentors. The Village also has a pre-accelerator program that works with minorities and women in the early stages of their business development.
Accommodations within walking distance of ATV start at $108 nightly.
SpringHill Suites by Marriott Atlanta Buckhead $143
Hampton Inn Atlanta-Buckhead $108
Element Atlanta Buckhead $181
Courtyard by Marriott Atlanta Buckhead $142
Embassy Suites by Hilton Atlanta Buckhead $179
Grand Hyatt Atlanta In Buckhead $310
W Atlanta – Buckhead $274
Buckhead is easily accessible by train cab, or Uber from Atlanta’s Hartsfield-Jackson International Airport.
Hartsfield-Jackson serves 150 U.S. destinations and more than 75 international destinations in 50 countries.
Early Full Registration: $449
Regular Full Registration: $499
Single Day Registration: $299
IMPACT24 Program
9:00 AM – 10:00 AM ET
Keynote Presentation
10:00 AM – 12:00 PM ET
Breakout Sessions and Tech Demos
12:00 PM – 1:00 PM ET
Lunch with Exhibitors
1:00 PM – 3:30 PM ET
Breakout Sessions and Tech Demos
4:30 PM – 6:00 PM ET
Meetup Reception
9:00 AM – 10:00 AM ET
Keynote Presentation
10:00 AM – 12:00 PM ET
Breakout Sessions and Tech Demos
12:00 PM – 1:00 PM ET
Lunch with Exhibitors
1:00 PM – 3:00 PM ET
Breakout Sessions and Tech Demos
3:00 PM ET
Concluding Session
For over 45 years, CMG has been, and continues to be, the source for vendor-agnostic expert advice and information for IT professionals dedicated to managing the enterprise’s hardware, software, infrastructure, and computing systems across the enterprise.
CMG is now accepting session proposals for our 2024 IMPACT conference, an in-person conference opportunity. We’re looking for 30 to 40-minute sessions on these key topics to be submitted by November 10th.
- Cloud
- Platform Engineering / Containers
- Risk Management
- AIOps
- Performance and Capacity
- Engineering/DevOps/CICD
- Modernization
- AI/ML
- Security and Observability
- Edge Computing
GOOD VIBES
Feel the spirit
event schedule
- First Day - Feb 6, 2023
- Second Day - Feb 7, 2023
- Third Day - Feb 8, 2023
Time
Events
Speakers
- 10:00 AM - 11:00 AM ET
- Virtual
Opening Remarks Keynote Presentation: Increase the Productivity of PC Super Users
- 11:00 AM - 12:00 PM ET
- Virtual
- 11:00 AM - 11:30 PM ET
- Virtual
- 11:00 AM - 12:00 PM ET
- Virtual
- 11:30 AM - 12:00 PM ET
- Virtual
- 12:00 PM - 1:00 PM ET
- Virtual
Chenhua Shi, Data Scientist, Ericsson
- 12:00 PM - 1:00 PM ET
- Virtual
- 12:00 PM - 1:00 PM ET
- Virtual
- 01:00 PM - 02:00 PM ET
- Virtual
Time
Events
Speakers
- 10:00 AM - 11:00 AM ET
- Virtual
Broadcom Mainframe Software
- 11:00 AM - 11:30 AM ET
- Virtual
IMPACT Innovation Awards Presentation
- 11:30 AM - 12:00 PM ET
- Virtual
- 11:30 AM - 12:00 PM ET
- Virtual
- 11:30 AM - 12:15 PM ET
- Virtual
- 12:00 PM - 12:45 PM ET
- Virtual
- 12:00 PM - 12:45 PM ET
- Virtual
Arvid Trubin, Python Developer, Trutech Development, LLC
- 12:15 PM - 12:45 PM ET
- Virtual
- 1:00 PM - 1:30 PM ET
- Virtual
- 1:00 PM - 2:00 PM ET
- Virtual
Giordano Sannino, Junior System Programmer, Sogei
- 1:30 PM - 2:00 PM ET
- Virtual
Time
Events
Speakers
- 10:00 AM - 10:15 AM ET
- Virtual
- 11:00 AM - 11:30 AM ET
- Virtual
- 11:00 AM - 12:00 PM ET
- Virtual
- 11:00 AM - 12:00 PM ET
- Virtual
- 11:30 AM - 12:00 PM ET
- Virtual
- 12:00 PM - 12:45 PM ET
- Virtual
- 12:00 PM - 12:45 PM ET
- Virtual
Nicole Camara, Product Manager, IBM
- 1:00 PM - 2:00 PM ET
- Virtual
- 1:00 PM - 2:00 PM ET
- Virtual
- 1:00 PM - 2:00 PM ET
- Virtual
Dr. Alex Lupersolsky, CTO, BEZNext
Get Tickets
Regular Plan
$99
250/500
- Concert Attendance
- 20 Lottery Ticket
- Priority Seating
- 5 Person Entry
- Certificate
- T-Shirt
Business Plan
$399
200/500
- Concert Attendance
- 20 Lottery Ticket
- Priority Seating
- 5 Person Entry
- Certificate
- T-Shirt
Enterprise Plan
$499
50/500
- Concert Attendance
- 20 Lottery Ticket
- Priority Seating
- 5 Person Entry
- Certificate
- T-Shirt